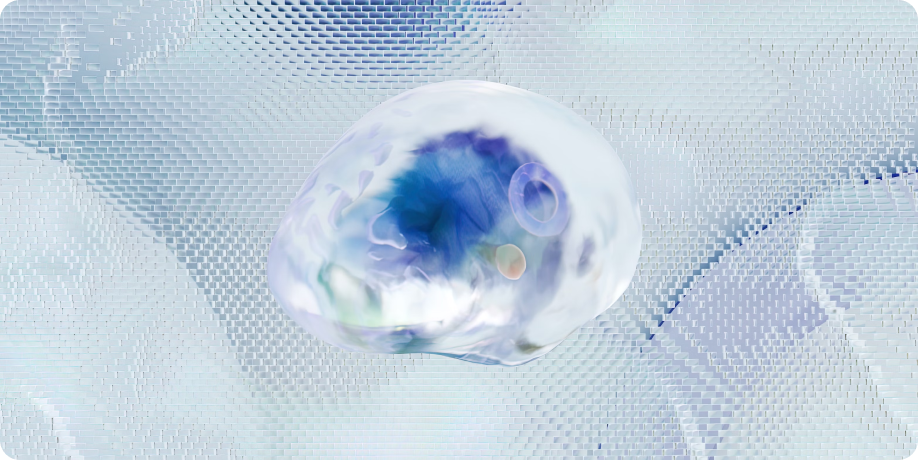
Every company is a data company – or at least they could be.
Just as the rise of software forced major reckonings in every industry that ultimately led nearly all companies to add deep technical competencies in order to survive, large and small organizations alike are taking the next step along this path by learning to make the most of their first-party data assets – that is, the data they’re collecting directly from their customers or users. Still, when it comes to getting value from customer data assets, licensing that data for use by partner organizations is often overlooked. The barriers to entry are high, requiring proper strategy, ongoing effort, and sometimes even dedicated tooling, all of which can be meaningful distractions from a business’s core objectives.
That won’t be the case forever, though. Consumers want more than just better protection for their data; they want ownership and control – and they’ll get it one way or another. Such co-ownership will bring about seismic changes, including the rise of customers looking to monetize their own data in partnership with the organizations that collected it. For this to work, businesses will need to think carefully about the assets they have and how they might be deployed. Unsure of where to start? Read on.
1. Map Your Data
The first step in developing a data monetization strategy is to assess the data that you’re collecting. This will fall into a number categories, but we’re primarily going to focus on data that can be tied to individual customers rather than general metrics from within your business. That said, there’s no universal customer data taxonomy, so it’s best to start thinking in broad terms like the following:
Transactional data: If you sell something to your customers – even if it’s only a web subscription – those transactions are generating potentially useful information. Purchase history, the price of individual products, categories shopped, precise date and time of sales, and more contribute to building a picture of who customers are and what they prefer.
Identity assets/demographic data: This bucket includes most of the things that leap to mind when thinking about “customer data.” It includes things like first and last name, address, phone number, age, gender, and so on. Some industries will have access to even more information that may be relevant in-context, such as education, marital status, or income level.
Behavioral data: At the highest level, behavioral data describes how users interact with your various digital (and even sometimes physical) touchpoints both before and after transacting. Pages browsed (and for how long), buttons clicked, articles read, shopping carts created and abandoned, and similar activities all constitute behavioral data.
The important thing is to be thorough: identify what you have, how it’s collected, and what system (or systems) of record store it. Your marketing team will be immensely helpful here.
Once you’ve identified your discrete customer data assets, the next step is to combine them in order to create derived customer data. Derived data can be simple, such as using an orders table to derive a customer’s average order value or total lifetime value. It could be vastly more complex, such as surfacing a segment of high-value customers who live in the upper midwest and have spent over a given threshold of money on a specific item category – the more creative you are, the more precise you can be. Once again, it’s likely that your marketing team will have notions of advanced segmentation that could be of use here, so follow their lead.
2. Define Value Hypotheses
By now you’ve pulled together quite a bit of useful information about your customers: you know who they are, what they’ve bought, and how they’ve interacted with your digital estate, and you’ve enhanced this understanding by thinking through novel combinations of those attributes, transactions, and behaviors that reveal new and interesting insights. It’s now time to formulate some hypotheses around how this data might be valuable to other organizations – in other words, your potential data buyers.
This part of the process can be daunting, but it’s not your job to come up with every possible nuanced use case for businesses that aren’t yours. It’s simpler and more effective to think in terms of where the value hypothesis originates, whether it’s internal to your organization, with a known partner, or by someone else entirely:
Direct-to-partner: These are the most straightforward use cases because they originate from 1:1 dialog with a partner organization, typically one with shared customers, a related product, or in an adjacent industry. In fact, it’s common for data partnerships to emerge from strategic go-to-market alliances. However they arise, the organizational affinity and direct conversations driving these relationships allow for direct, iterative discussion around the partner’s data needs.
Moving beyond 1:1 data partnerships, you’ll most likely take advantage of some kind of data marketplace. This means packaging your data such that it’s easy for unknown third parties to evaluate whether or not they’d like to buy it:
Curated: These are known (or mostly known) use cases facilitated by your data assets, and in many cases they will closely resemble the kinds of use cases you encounter with known partners. Audience is key here: think about how you might bundle your data points to make them attractive to buyers within a given industry looking to gain insights, improve sales, or target potential customers more effectively.
Emergent: This bucket is the least explicitly defined because it speaks to the emergent, unplanned value of your data assets. While you’ve ideally made significant progress identifying direct partners and creating sound theories of value for your assumed buying audience, there’s nevertheless a long tail – or potentially significant untapped markets – of potential buyers who might find any number of unexpected uses for your data. You can’t anticipate these use cases by definition, so focus instead on ensuring that your data offerings are sufficiently diverse and granular to allow for novel uses down the road.
Don’t feel pressure to get everything right on the first try. Your overall data strategy, and therefore this sort of exercise, should be in a state of constant iteration and refinement. Remain thoughtful, be persistent, and incorporate new information into your approach as it becomes available.
3. Take Compliance Seriously
With much of the heavy strategic lifting out of the way, you’re free to toss your customer data into a marketplace, sit back, and watch the sales roll in…right? Fortunately for your customers, not quite. It’s crucial to adopt a data governance strategy that accounts for data regulatory frameworks such as GDPR, PIPEDA, and the growing web of legislation emerging from individual US states. In short, you must confirm that you have the right to sell your data and that your potential buyers have the right to access it, which typically means restricting or anonymizing portions of your dataset.
This process can sometimes lead to disappointment. You can have any amazing value hypothesis – or even confirmed intent and willingness to pay on the part of buyers – about some of your data assets that you simply can’t monetize, and at that point the only option is to walk away. Not only is the legal and reputational risk too great, it’s also a violation of the trust your customers have placed in you.
Of course, the above is only true based on the system as it exists today. As noted at the beginning of this piece, your customers are clamoring for meaningful control of their data that goes well beyond “opt-in/opt-out,” and you should be just as eager to give it to them because you can enlist them as your data monetization partners. If you can find ways to bring them into the process and obtain their affirmative, ongoing consent, you will find yourself in position to offer incredibly rich, deterministic data assets for sale that can’t be matched elsewhere, and both you and your customers will benefit greatly from that.
Precisely how your organization achieves this is up to you, but it will require a great deal of customer education, not to mention overcoming a number of technical hurdles, which is why we’re building SELF. Our vision is to create the world’s most valuable, most secure, most egalitarian data marketplace wherein all participants reap the rewards.
Keep an eye on this space for more information as it’s made available, and in the meantime you can prepare by getting a head start on your data monetization strategy today.